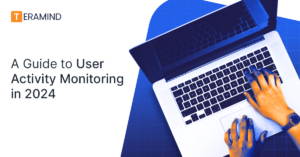
Workforce analytics software collects, analyzes, and visualizes employee data to help companies make data-driven decisions about their workforce. With hundreds of different workforce analytics tools currently...
Manage insider risk, optimize productivity, and enforce compliance with Teramind.
Solutions
Cybersecurity
Productivity
New From Teramind
Company
Insights
Explore Insights
Partners
Solutions
Productivity
Cybersecurity
Advanced
Insights
Resources
Teramind Labs
Company
© 2014-2024 Teramind Inc.